Mastering a composable customer data strategy with a data warehouse and CDP
When it comes to customer data, your organization has likely significantly invested in centralizing it — likely by using a cloud data warehouse like Snowflake.
While this has yielded clear benefits in areas like data analytics and business intelligence, other departments, like marketing, are still struggling to realize the full value of this investment.
Despite adopting best-of-breed tools, your MarTech team faces integration challenges and untapped potential in customer data utilization. Additionally, there’s still “dark data” outside of your warehouse, representing critical information about customer engagement and your marketing efforts.
This article introduces the concept of data composability as a solution to these challenges. We’ll explore how a composable data strategy differs from traditional ones, the key aspects of a successful composable data strategy,
Traditional data strategies vs. composable data strategies
A traditional data strategy centers on centralizing and managing customer data. It establishes best practices around governance, architecture, management, and quality, focusing primarily on the process of getting data into a central location.
However, it often places less emphasis on how your organization will unlock value from the data once it’s centralized.
In contrast, a composable data strategy is driven by what you will do with your data. The primary goal of creating and modeling data is to power downstream applications.
While you likely have a clear understanding of your analytical and predictive use cases, the data needs for CDP use cases — such as customer retention in marketing, acquisition in advertising, customer relationship management, and customer support — might not be as well-defined.
Key pillars of a composable data strategy
There are four key pillars of a composable data strategy:
- Data creation
- Data centralization
- Data modeling
- Data applications
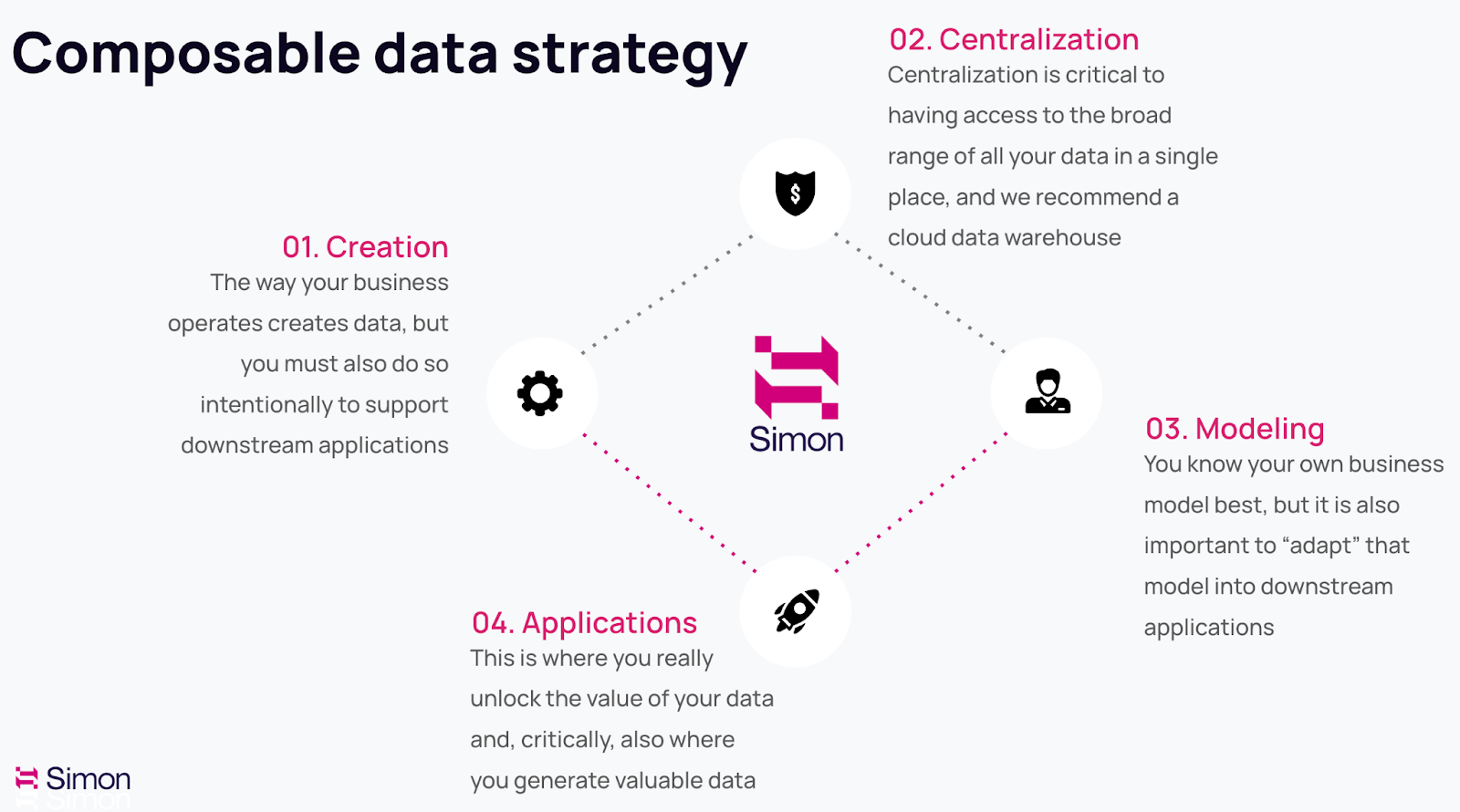
Let’s dive into each pillar.
Customer data creation
While not the central focus of a CDP like Simon Data, data creation is critical. Instead of treating data as mere “exhaust” from business operations, we need to align data creation with CDP requirements.
The Simon team can clarify these requirements, offer out-of-the-box data creation capabilities, and recommend best-in-class partners, like Snowplow, to optimize this stage of the data strategy.
Data centralization
Centralizing your data is about more than just moving it into a cloud data warehouse like Snowflake. It’s about ensuring that all relevant data — whether customer behavior, transactional records, or marketing interactions — is aggregated in a way that supports your broader data strategy.
This centralization enables better data governance, easier access, and a stronger foundation for downstream applications.
Data modeling
Data modeling involves two key components:
- Organization-wide domain model
- Specific customer model for your CDP
The challenge is creating a data model that acts as a compatible “adapter” between your complex business model and the focused CDP model. This enables your CDP to support high-value data applications without being locked into a rigid or incompatible framework.
Applications
The real power of a composable CDP emerges when you apply your data. These applications span retention, acquisition, relationship management, and support.
This composable approach allows you to adapt your data model to fit specific needs while feeding generated insights back into your data creation and centralization processes.
Why a composable data strategy and composable CDP work
You typically create, centralize, and model data on your own. However, a composable CDP plays a crucial role in adapting your data model to meet the specific needs of high-value applications.

It also ensures that valuable data from these applications is captured and fed back into your data creation process — data that would otherwise be missed without a CDP integrating your marketing, advertising, and support teams into your overall data strategy.
Data interoperability
Now, let’s discuss data interoperability — how data is exchanged, communicated, and used between systems — between traditional data workflows and composable ones.
Raw data alone cannot power meaningful use cases; it needs to be adapted for downstream applications.
Data interopability in a traditional CDP vs. a composable CDP
In a traditional CDP, this modeling work is typically done only after exporting data into the CDP itself, making it specific to CDP use cases and effectively “locking in” that data, preventing it from being used beyond the CDP.
Data that flows into the CDP includes:
- Raw events: Capture real-time actions and behaviors of users across various touch points
- OLTP data: Includes critical transactional and user data, such as purchases, account details, and user interactions
- Product catalogs: Information about the products or services your organization offers, including inventory levels, descriptions, and pricing
- Fulfillment data: Tracks the status of orders, deliveries, and any logistics-related information
- Scored predictive models: Pre-computed insights and predictions, such as customer lifetime value or churn risk, help with segmentation and personalization
Data flowing back to the CDW includes:
- Segment membership: Information on which users belong to specific segments based on behaviors, attributes, or predictive scores
- Personalization: Data related to personalized content, product recommendations, and messaging tailored to individual users
- Campaign metadata and execution: Details about marketing campaigns, including objectives, targeting criteria, and execution data, such as send times and delivery status
- Downstream channel engagement: Data capturing how users interact with marketing efforts across channels, including email opens, ad clicks, and website interactions
But in a composable CDP, all data is in the CDW. This includes:
- Unified data hub: All data, whether it’s incoming raw events or outgoing segment memberships, remains centralized within the cloud data warehouse
- Flexible utilization: Because all data is housed in the CDW, it’s not locked into specific applications or use cases. It’s adaptable and accessible for a wide range of applications — from CDP use cases to advanced analytics, artificial intelligence, machine learning, and beyond
- Seamless integration: With Simon’s composable architecture, the modeling and enrichment work happens in the CDW, enabling the same datasets to power various applications without duplicating effort or data
The differentiated value of Simon’s composable architecture lies in its flexibility: you can perform all of this modeling work within the CDW. The benefit? You can leverage your data not only for CDP use cases but also for broader organizational needs, breaking free from the limitations of traditional CDPs.
The most important aspect of a composable data strategy: data applications
Let’s address the most critical aspect of a composable data strategy: the data applications you need to unlock with the data you’ve centralized within the cloud data warehouse.
As I said earlier, some data applications are more immediately accessible, given the state of the tooling ecosystem around the CDW and your organization's traditional team structure and skills composition.
For instance, your data science team is likely deeply embedded in your Snowflake instance. They’re not only directly accessing your data through SQL, but their traditional workbench of Jupyter notebooks controlling and evaluating predictive models, seamlessly slots into the CDW via capabilities like Python for Snowpark and Snowflake’s baked-in support of tools like notebooks and DataFrames.
You’ve likely already given them deep access to Snowflake, a computing budget, and perhaps even governance training and specific tooling workshops to further enable their interaction with your data.
Beyond BI and predictive modeling, there are additional high-value applications that are crucial to a composable data strategy. These applications often fall under the purview of marketing, advertising, and customer engagement teams.
- Segmentation: Create precise customer segments based on centralized data, enabling targeted marketing efforts that resonate with specific audiences. Segmentation within a composable CDP ensures consistency across all platforms and channels.
- Personalization: Deliver personalized experiences at scale by utilizing customer data stored in your CDW. Personalization is driven by accurate, up-to-date profiles that reflect the latest customer interactions, ensuring relevance and engagement.
- Activation: Activate customer segments by syncing them with various marketing channels, ensuring that campaigns are data-driven and optimized for performance. Activation leverages the CDW's real-time capabilities, ensuring that data is fresh and actionable.
- Orchestration: Coordinate complex, multi-channel customer journeys that are responsive to real-time data. Orchestration within a composable CDP ensures that all customer interactions are harmonized, creating a seamless experience across touch points.
The future of customer data management is composable
As organizations look to maximize the value of their data investments across departments like data, marketing, and sales, data teams need to adopt a composable data strategy.
By leveraging a cloud data warehouse like Snowflake and a composable CDP like Simon Data, data teams can unlock the full potential of its customer data.
A composable data approach allows you to:
- Unify your data in a central hub
- Adapt your data model flexibly to various use cases
- Power high-value applications across your organization
- Ensure consistent data utilization across all teams and tools
The result? A more agile, efficient, and effective data ecosystem that drives real business outcomes. But remember that a composable data strategy is not just about technology. It's about aligning it with your business goals, breaking down silos, and fostering a data-driven culture across your organization to drive the ultimate customer experience.