Harnessing predictive analytics for smarter marketing
As marketers, it can be hard to take a breath and plan for the future. You may be stuck putting daily fires out, so when do you have time left to strategize?
Predictive analytics can help you build a forecast and devise a marketing plan. It saves you from wading through spreadsheets and finding the patterns and trends yourself — and with advancements in AI and ML, predictive analytics are only getting better and easier to implement.
What are predictive analytics?
As the name suggests, predictive analytics use data modeling to predict the future.
If you’ve used past KPI benchmarks, market conditions, and competitor research to triangulate how you’ll perform this quarter, congrats! That’s a prediction. You took data from multiple sources to make an inference.
Where predictive analytics models improve on this technique, however, is in the scope with which they’re able to perform their modeling. Predictive analytics uses data mining, machine learning (ML), modeling, and the latest AI advancements to create predictions. Some models can give you this data in real time.
You‘re probably already connecting the dots and seeing why this is helpful, but let’s go over why predictive analytics can help you execute marketing strategies that create a personalized customer experience.
Why are predictive analytics helpful for marketers?
Marketers use predictive analytics for all sorts of things (and who doesn’t love a multitool?). They have some key benefits that address most marketers’ pain points:
Less time wading through customer data
Using predictive modeling saves time. Rather than having a human manually gather data from multiple sources — especially from data engineers if you don’t use a cloud data warehouse or a customer data platform — and put together a report (let alone do this regularly), predictive modeling can source insights for you.
Many marketers struggle to find time to gather the right customer data to adapt to customer needs and analyze marketing campaign metrics in real time, so it makes sense to offload this task to a trusted tool to do this for you.
Better data-rich insights
Once you’ve set up your project, predictive modeling pulls from multiple sources (typically sources aggregated in a cloud data platform, such as Snowflake, and activating that data customer data platform (CDP) such as Simon) to power up your decisions with data. Backing your ideas up with the customer data you already have leads to faster action, easy marketing campaign implementation, and real results.
Real-time updates
Trends move fast. Have you ever meticulously planned a campaign only to see the cultural zeitgeist shift before execution? Or try to send out a personalized SMS discount, only to learn the customer prefers emails?
Predictive analytics give you the most accurate customer data when you need it — and as customers’ needs change — to help you strategize the most effective marketing plan quickly and easily.
Are you sold on using your data for better predictions? Then let’s walk through some use cases to activate your data.
How to use marketing predictive analytics for actionable insights
When it comes to marketing, you can use predictive analytics just about anywhere — after all, you can interpret and assort data for almost any use case. To narrow it down, these are some of the big ones for more effective marketing strategies.
Segmenting audiences
With some handy dandy machine learning, your predictive modeling can use cluster modeling to group users into buckets, typically by:
- Geographic segmentation
- Psychographic segmentation
- Demographic segmentation
- Behavioral segmentation
- Other, such as lifecycle, buyer’s journey stage, potential churners, persona, etc.
Once you’ve segmented these audiences, you can activate your data by sending targeted campaigns to the right people on the right channel, at the right time.
Potential buyers
If you’re looking for a way to pinpoint potential customers, identification modeling can use existing data on your customers to pinpoint users like them who are close to a purchasing decision.
Simon Data has an example of this: purchase propensity. Being a CDP that integrates with Snowflake, Simon uses machine learning to find contacts that are primed for conversion — and contacts that are on the fence about buying.

Another example of this is lead scoring. Predictive analytics can rank prospects by the possibility of conversion based on the prospects you already have. This is a nifty way to determine if a lead is qualified, or if your marketing efforts are surfacing qualified leads to begin with.
Potential churners and cart abandonment
Wouldn’t it be nice to catch customers before they churn and send them an email with an incentive to renew? Or, maybe you want to catch a group of people who aren’t able to fill out your checkout form and complete a purchase. Predictive analytics smartly identifies commonalities between your churners and can predict those likely to churn.
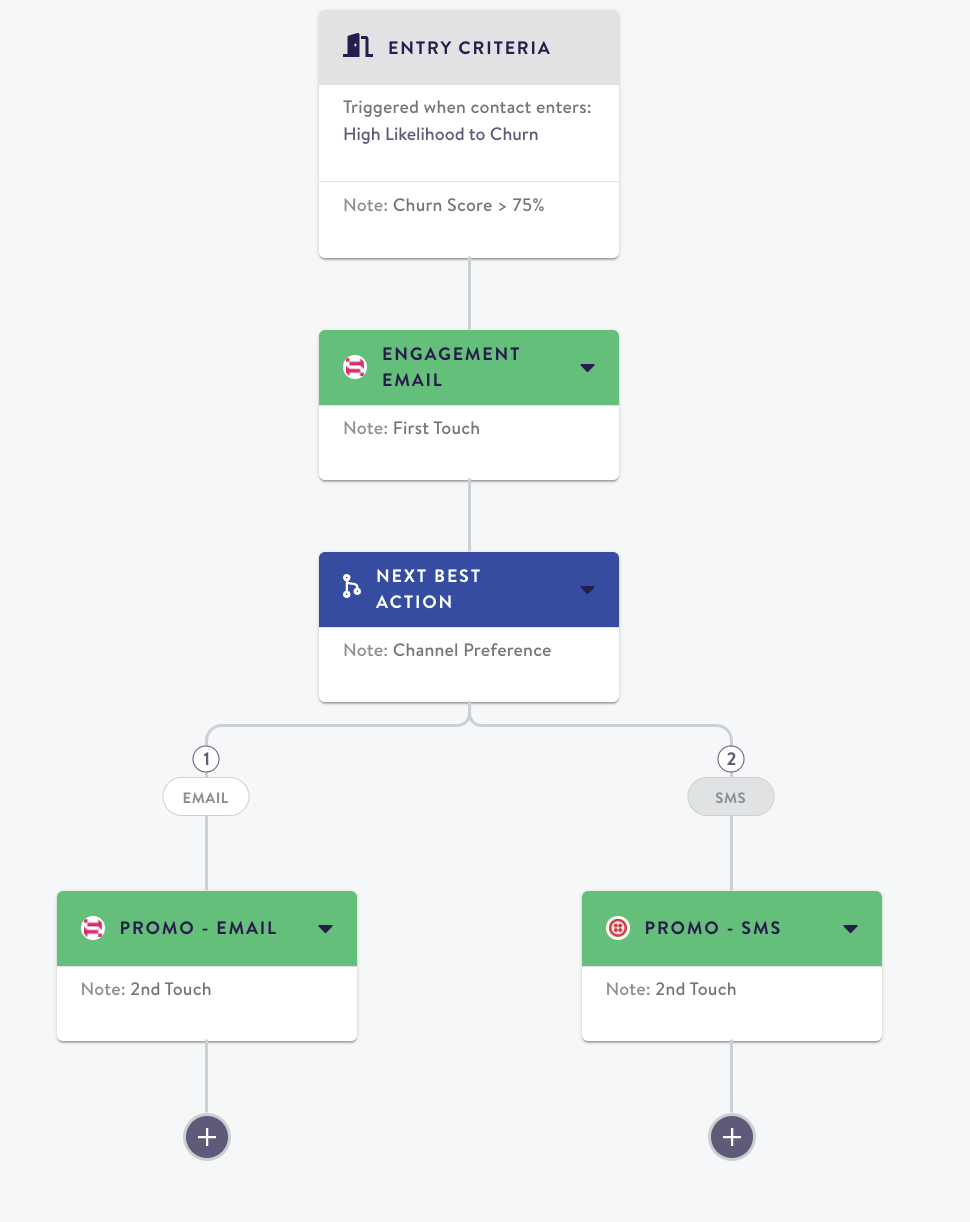
For instance, Vimeo uses segmentation to solve a big problem: free users churning before they uploaded their first video. Vimeo fine-tuned their email reminders to focus on users who abandoned their upload in real time. In this case, Vimeo was already aware free users were most likely to churn if they didn’t upload a video in their first seven days, but predictive analytics could also surface this issue.
Potential remarketing opportunities
Predictive analytics can find a segment of website lurkers. Better yet, you can also target users who are most likely to make a second purchase, or offer personalized discounts to incentivize customers who need a nudge.
Barkbox uses a CDP to run complex A/B tests and gauge whether their discounts are effective. Predictive analytics can take this one step further, finding users who’d be likely to purchase again if you offered a discount.
Staying on top of trends
With real-time data sourced from a wealth of customer information, you can predict and plan for trends faster than anyone else in the industry. Machine learning surfaces these trends from your data and gives you a chance to plan ahead.
For example, your predictive model may pick up on a seasonal downturn that will impact your industry based on the historical data it has. From this, you can prepare to weather the storm, as well as have a better understanding of why sales may be lower in this upcoming seasonal downturn.
Better customer personalization
The better you know your users, the more personalized your content can be. And personalization makes a big difference these days in how customers interact with brands.
Take as an example an industry that needs to be highly personalized: luxury travel. Travel + Leisure Co. successfully uses predictive analytics to offer vacation recommendations by gauging customers’ purchase propensity, perceived value, and urgency.
As you can imagine, this makes a huge difference over travel sites that serve the same vacation packages to everyone, and even more so for a customer searching for a luxury experience expecting to pay top dollar.
Say a luxury travel site might see a user returning over months, lowering the chance there’s a high level of urgency for their purchase. However, the user might have looked at packages to Fiji several times, thus increasing their purchase propensity.
And let’s say these packages are expensive, ranking their value highly. It makes sense that the travel company serves up targeted content (travel information, guides, discounts, etc.) to its relevant audience about trips to Fiji, with a departure date that’s not urgent. If you were that customer, you’d probably prefer this personalization.
Machine learning and AI make predictive analytics tick
With these facts in mind, wouldn’t anyone want to use predictive analytics? Obviously. If it came free, and if it were so easy to implement. The challenge is having a team with the skills to create an advanced predictive model, and the ability to house and activate your data in one hub.
That’s why most of us outsource this complex data modeling to a third party. CDPs can empower you to activate customer data if you’re storing it in a warehouse. The best CDP choices for predictive analytics have AI and machine learning with the ability to deliver these insights in clear, actionable terms.
With technology so new, you can be sure of a competitive edge. Not many tools offer predictive insights packaged neatly, but if you’re interested in finding one, consider booking a demo with Simon Data to learn more about its predictive features.