Snowflake Arctic: Changing the climate of data-driven marketing
You may have seen Snowflake’s major launch of their proprietary LLM called Arctic yesterday. And if you thought to yourself, “Snooze, yet another LLM that’s competitive with industry benchmarks,” you’d be joined by others, such as TechCrunch, who had similar reactions.
But TechCrunch is dead wrong.
Under the hood, the technology behind Arctic is incredibly impressive. The model has 480 billion parameters, training is optimized by a “Mixture of Experts” (MOE) architecture, and it features a three-part learning curriculum to optimize enterprise intelligence. Snowflake’s press release is incredibly detailed and goes into extraordinary depth on how it works.
This stuff is all super cool, and the technology itself is also somewhat inaccessible — even for someone like myself with a machine learning PhD!
But let’s take a step back and look at the larger picture. This launch effectively propels Snowflake as a key player in the LLM race, an area where they had been lagging for over a year. For Snowflake, it’s not about building a model with 500B parameters, it’s about developing a complete set of capabilities required to drive AI adoption for business applications. The algorithm is only a small piece of the puzzle.
For Snowflake, it’s not about building a model with 500B parameters, it’s about developing a complete set of capabilities required to drive AI adoption for business applications.
With Arctic now in place, Snowflake is poised to affect massive disruption around business processes and drive huge innovation around how LLMs can deploy in an enterprise setting.
Starting simple with AI
Let’s pretend you’re selling socks on the web. Today, you can go to OpenAI right now and ask the following:
>> Write me an email encouraging a customer to buy a pair of socks
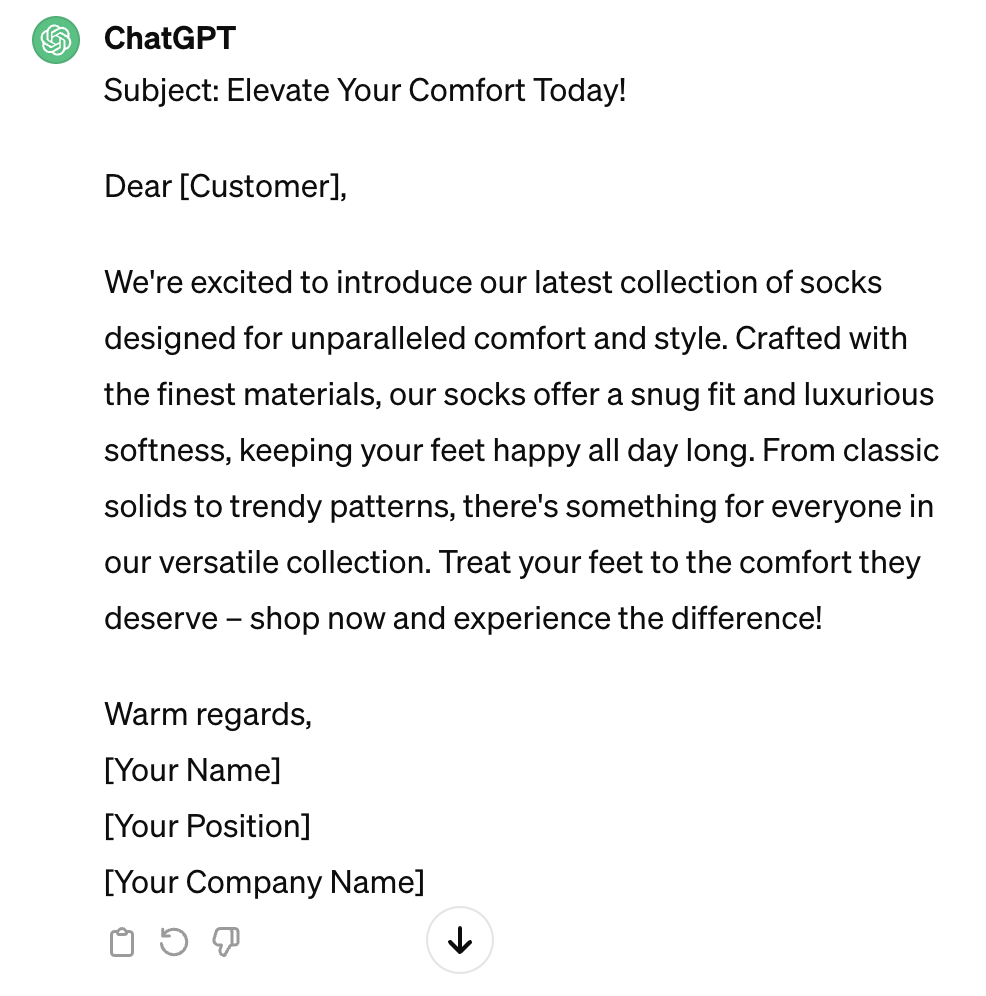
While the message is well-written, clear, and to the point, it’s not personalized, it’s not on-brand, and it has no context of historical effectiveness and what’s required to drive engagement and conversions. It’s also boring and sounds like something a first-year marketing student would write.
With a bit of information about the recipient, you can iterate on this significantly:
>> Now assume the customer is training for a marathon and likes to wear vivid colors
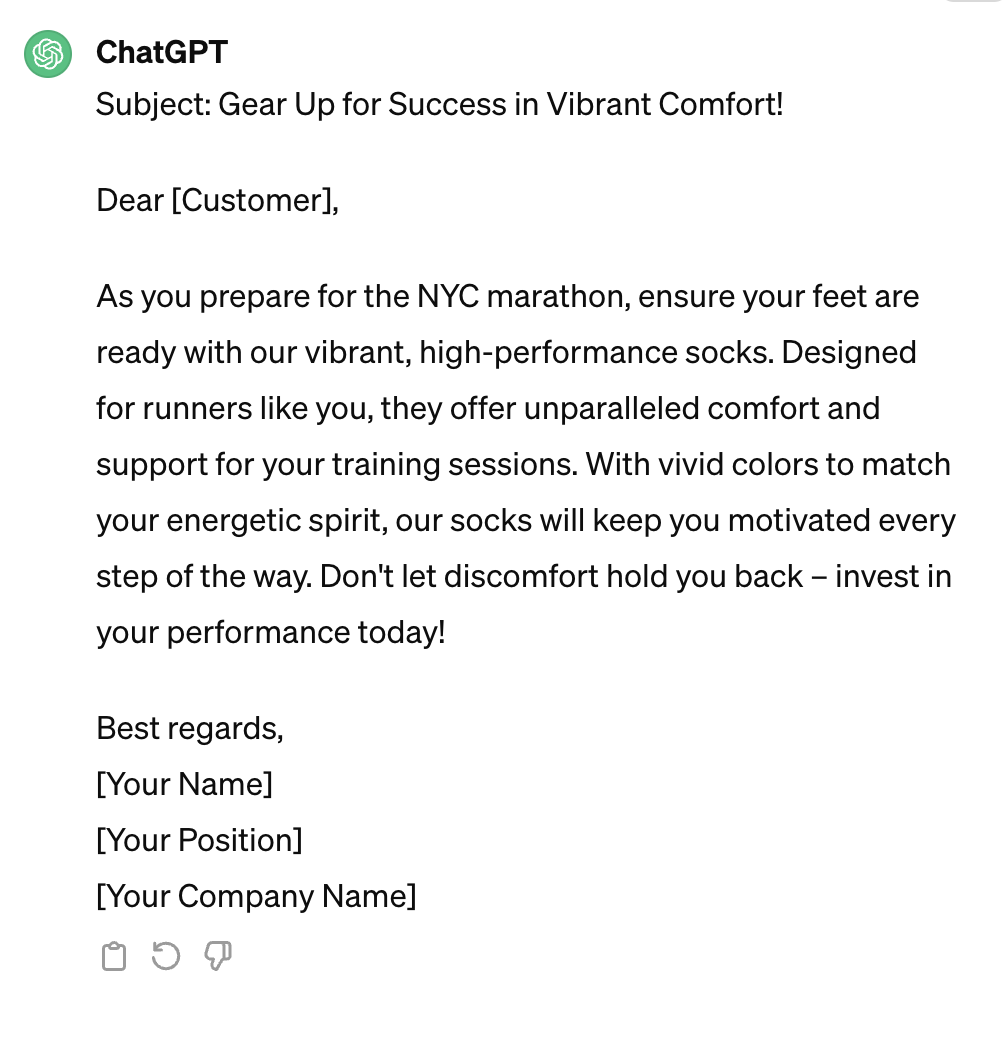
While this is an improvement, the message lacks a brand-specific tone and is built with zero insight into what sort of messaging is effective in converting sales.
Taking a step back, use cases like things generally require a set of basic enterprise requirements to truly be successful.
Requirement 1: High-quality data
There’s an adage in data: “Garbage in, garbage out,” and if the context on the customer is inaccurate or incomplete, algorithm quality just doesn’t matter. Understanding customer preference, real-time behavior, and historical content engagement is critical for success.
In this example, drawing from past messages written by the brand about similar topics, correlating that with historical engagement and conversion rates, and then pairing it with relevant customer data are some of the key data elements required for success.
Requirement 2: High-quality algorithms
The LLM needs to work for the application and use case, and in this example, there are real challenges around matching brand tone with compelling calls to action to drive outcomes. Without getting too much into details, optimizing context windows can be difficult in use cases like this, so the ability to tune or retrain models to fit brand voice is critical.
Requirement 3: Business application support
It’s going to be quite some time before marketing gets completely automated away 🤯. Until then (read: in my lifetime), tightly integrated applications are required to guide the algorithm, activate the outputs into the end use cases, and then experiment and iterate to learn what works and what doesn’t. An effective application strategy is much more than a pipe for execution. Rather, it creates a tight feedback cycle to drive business outcomes.
Requirement 4: Fully secure and governed
Your customer data is the crown jewel of your business. Data security today is more critical than ever — and your customers expect this more than ever.
The mad enterprise scramble
Ever since OpenAI launched in November 2022, there’s been a mad scramble across players to capitalize on opportunities like the use case outlined above.
In Salesforce’s most recent earnings call, Marc Benioff spent the majority of the time addressing Salesforce’s new Data Cloud offering alongside their AI offering, and spoke directly about this opportunity:
“Put your hand up if you're using Snowflake every day…. So many of our customers have islands of trapped data in all of these systems, but the AI is not going to work because it needs to have the seamless amalgamated data experience of data and metadata, and that's why our Data Cloud is like a rocket ship.”
Salesforce is masterful in identifying and positioning high-level business problems, yet their company DNA is rooted in software challenges that are far less technical than the challenges that modern cloud data and LLM-based AI have presented in recent years.
If I were a Fortune 500 CIO, I would not select Salesforce’s Data Cloud as the focal point of a five-year data strategy, if nothing but for the fact that Salesforce is 10 years late to this market.
What Snowflake has uniquely accomplished with the launch of Arctic is a completion of the value triangle:
- Data quality: Snowflake has been an established leader here for quite some time. Industry benchmarks across performance, cost, and scalability have consistently demonstrated this, and the scale at which Snowflake deployed also shows clear success.
- Application support: Earlier this month, Snowflake launched its Marketing Data Cloud and featured a set of application partners that are purpose-built to deploy on top of Snowflake.
- AI support: Not only does Arctic make Snowflake a contender here, but they’ve been incredibly thoughtful around dimensions that matter for the enterprise. A focus on code generation provides huge opportunities for workflow automation that plagues so many marketers today. And training costs that are a fraction of existing solutions open a new world of opportunities to train on first-party data and content.
The beauty of this model is that all of these points are wrapped up in Snowflake’s dedication to end-to-end security, which has long been a focal point and a core value proposition for today’s CIO. Snowflake’s best-in-class tooling also comes with a next-generation of governance tools to fully secure your data while enabling data access and business outcomes.
What’s worth noting in all of this is that Snowflake is focusing on the foundational data components via its Cloud Data Platform strategy. Whereas Salesforce saw a gap and built its own data cloud, Snowflake is not seeking to build a complete set of martech applications (or any other category, for that matter).
For Snowflake, it’s about creating a secure platform with great data and, as of yesterday, great AI, to enable any application to be built to drive this next generation of use cases.